Understanding Medical Data Annotation: A Key to Healthcare Innovation
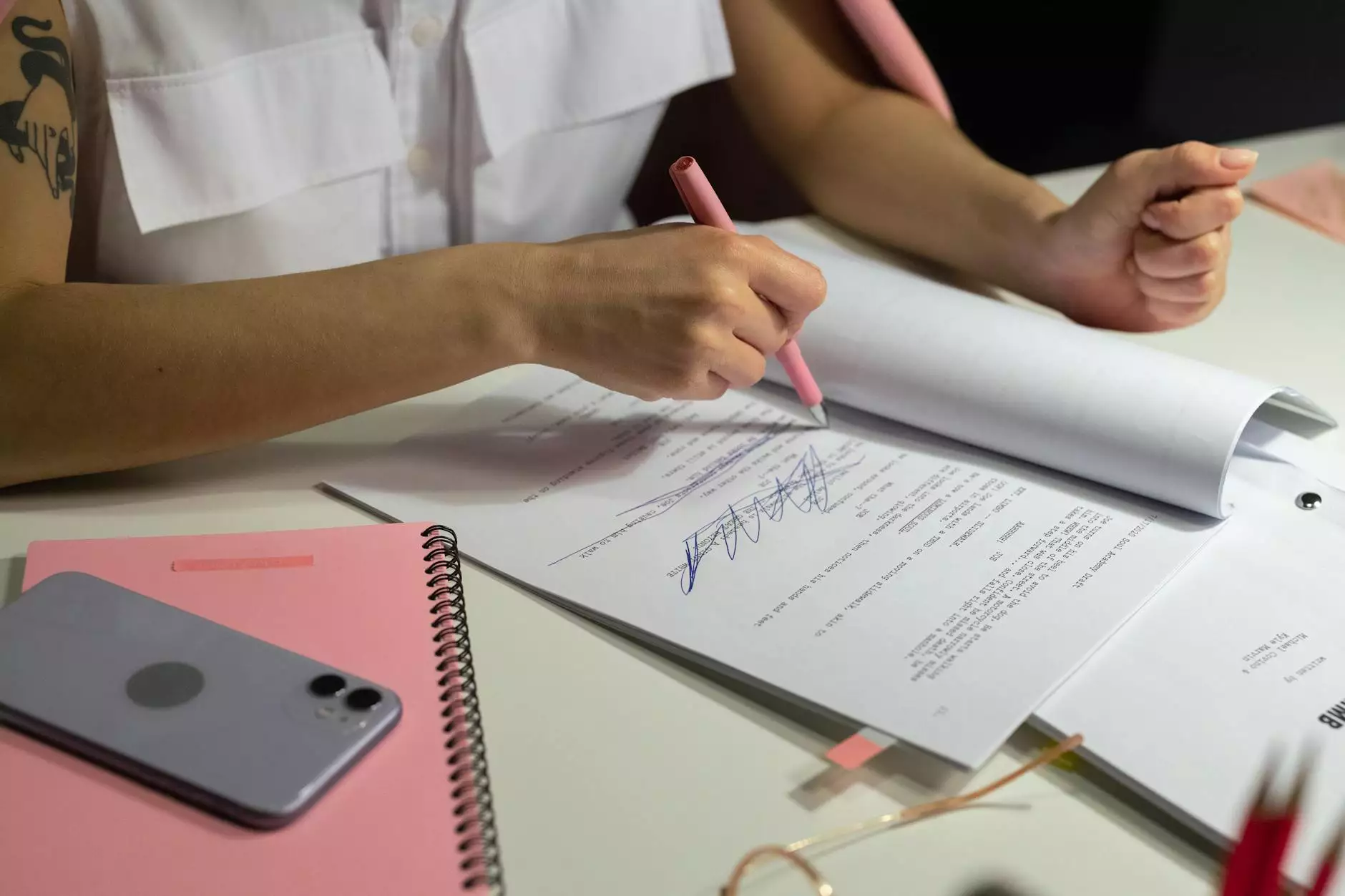
Medical data annotation has become an essential component of today’s healthcare landscape. As the medical field embraces advanced technologies such as artificial intelligence (AI) and machine learning (ML), the role of data annotation in streamlining processes and enhancing patient care cannot be overstated. In this article, we delve deep into what medical data annotation entails, its significance, best practices, and its impact on the healthcare industry.
What is Medical Data Annotation?
Medical data annotation refers to the process of labeling and categorizing medical data. This data can be in various forms, including images, videos, patient records, and clinical notes. The primary purpose of this annotation is to provide accurate context that machine learning models can interpret. For example, in medical imaging, annotators label specific features in an X-ray or MRI scan to train AI algorithms to detect anomalies or diseases.
The Importance of Medical Data Annotation
The application of medical data annotation can vastly improve healthcare delivery systems. Here are several reasons why it is indispensable:
- Enhanced Diagnostic Accuracy: Properly annotated data helps AI systems make precise diagnoses by training them on high-quality datasets.
- Streamlined Patient Care: Using annotated data, healthcare providers can optimize patient workflows and treatment plans.
- Improved Research Outcomes: Accurate data annotation enhances the efficiency of clinical trials and research studies, leading to better health outcomes.
- Automation of Routine Tasks: AI systems trained on annotated data can automate mundane tasks, allowing healthcare professionals to focus on patient care.
Types of Medical Data Requiring Annotation
Various types of medical data undergo the annotation process to ensure that AI models can understand and utilize them effectively. Some of these include:
1. Medical Imaging
Medical imaging refers to the visualization of the interior of a body for clinical analysis. Common imaging techniques include:
- X-rays
- Magnetic Resonance Imaging (MRI)
- Computed Tomography (CT) scans
- Ultrasound
In this context, medical data annotation involves marking specific areas of interest, abnormalities, or critical features within these images.
2. Electronic Health Records (EHR)
Electronic health records contain a wealth of patient data that require accurate tagging and classification. This includes:
- Patient demographics
- Clinical history
- Medication prescriptions
- Test results
Annotating this information facilitates data retrieval, improves accuracy in diagnoses, and supports research efforts.
3. Clinical Notes
Clinical notes written by healthcare professionals during or after patient consultations are often annotated for sentiment, relevance, and extraction of key medical information. This is crucial for:
- Natural Language Processing (NLP) systems that interpret human language.
- Predictive modeling to anticipate patient outcomes based on historical data.
Best Practices for Medical Data Annotation
Effective medical data annotation requires a structured approach to ensure accuracy and relevance. Below are key practices to consider:
1. Employ Expert Annotators
Utilizing professionals with domain knowledge, such as certified medical practitioners or trained medical coders, can significantly enhance the quality of annotations. They understand the nuances and context better than non-experts.
2. Ensure Consistency
Implement a clear set of guidelines and standards for annotators to follow. Consistency in labeling helps train AI models more effectively, resulting in improved reliability in AI outputs.
3. Utilize Advanced Tools
Invest in high-quality tools and software that facilitate seamless annotation processes. These tools often come equipped with functionalities that enhance productivity and accuracy.
4. Regular Audits and Quality Checks
Setting up regular quality checks and audits ensures adherence to annotation standards and allows for continuous improvement in the annotation process. Feedback loops are vital for maintaining quality control.
The Impact of Medical Data Annotation on Healthcare
The influence of medical data annotation extends beyond diagnostics; it revolutionizes various facets of healthcare:
1. Telemedicine and Remote Care
The growth of telemedicine relies on accurate data interpretation for virtual consultations. Annotated medical data supports telehealth platforms, allowing for rapid and reliable patient assessments.
2. Accelerated Drug Development
In pharmaceutical research, annotated datasets help in understanding drug interactions and potential side effects, thereby speeding up the development of new medications.
3. Personalized Medicine
By utilizing annotated data that captures patient-specific information and responses to treatments, healthcare providers can tailor personalized treatment plans that enhance patient well-being.
4. Predictive Analytics and Population Health Management
Annotated data plays a critical role in leveraging predictive analytics to forecast health trends. By analyzing large datasets, healthcare systems can allocate resources more effectively and manage population health proactively.
Conclusion
In summary, medical data annotation serves as the backbone of modern healthcare technology. As AI and machine learning continue to evolve, the need for quality annotated data will become even more crucial. By adhering to best practices and leveraging advanced tools, healthcare providers can ensure accuracy and efficiency in patient care, ultimately leading the way for a more robust and innovative healthcare system.
At Keymakr.com, we understand the significance of quality data annotation in healthcare. By providing tailored solutions and support, we help healthcare providers harness the potential of their data to improve outcomes and enhance patient care.